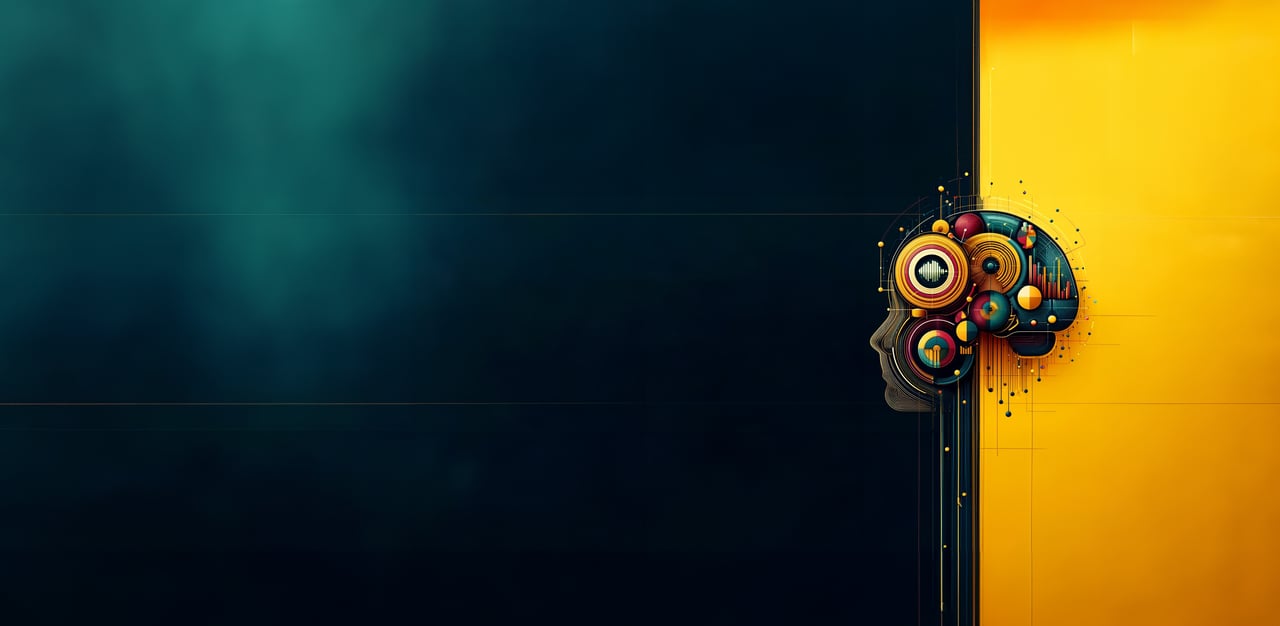
Strivenn Thinking
An AI Strategy is Now Essential
By Matt Wilkinson
The launch of DeepSeek sent shockwaves through the tech sector and sent consumers rushing to download the app. Concerns about data security, censorship and the code libraries the LLM prefers have followed the initial excitement about the launch.
The rush to adopt this lower-cost, yet highly efficient AI highlights a critical issue: without a structured approach to AI implementation, companies risk creating "secret cyborgs”: employees who unwittingly misuse AI, expose data to security vulnerabilities, and compromise ethical standards.
According to a recent report authored by consultants from McKinsey, employees are already three times more likely to already be using generative AI than their leaders expect. A well-crafted AI strategy should support this "bottom-up" approach to technology adoption and exploitation, within a robust risk management framework.
“The most effective AI strategies are deeply anchored in business objectives. Leaders who leverage AI to enhance organisational strengths to achieve business goals can and will drive real competitive advantage,” says Ram Viswanathan, transformation thought leader and Vice President of Strategy and Intelligent Automation at inGen Dynamics.
The Key Elements of a Strong AI Strategy
Below are the key elements that define a robust AI strategy.
1. Clear Objectives and Guiding Principles
A strong AI strategy begins with well-defined objectives that align with the organisation’s broader goals. These objectives may include driving innovation, improving operational efficiency, enhancing customer experience, or creating new revenue streams.
Beyond setting goals, organisations should adopt guiding principles that dictate the ethical and responsible use of AI. Principles such as transparency, fairness, accountability, and explainability ensure that AI systems remain understandable and aligned with organisational values.
2. Governance and Organisational Structure
Effective AI strategies require strong governance to provide oversight, mitigate risks, and ensure ethical compliance. This includes:
- Establishing AI Governance Committees: Organisations should form dedicated AI governance teams that oversee AI deployment, address ethical concerns, and ensure alignment with regulatory requirements.
- Cross-functional Collaboration: AI initiatives should not be siloed within IT or data science teams. Instead, they should involve legal, compliance, HR, and operational departments to ensure holistic oversight.
- Defined Roles and Responsibilities: Ensuring responsibility and accountability for AI initiatives across an organisation is critical – as is fostering an environment where controlled experimentation is encouraged.
3. Adoption of Strong Risk Management Practices
AI carries inherent risks, including bias, data privacy concerns, security vulnerabilities, and unintended consequences.
To mitigate these risks, organisations should implement robust risk management frameworks that include:
- Bias Detection and Mitigation: Regularly audit AI models for bias to ensure fair and unbiased decision-making.
- Data Privacy and Security Measures: Implement stringent data governance policies to protect sensitive information and comply with regulations such as GDPR and CCPA.
- Cybersecurity Considerations: The risks of data leaks and breaches have increased considerably, although implementing a robust AI strategy does help to mitigate many of the common pitfalls.
- Ethical Review Processes: Establish ethical review boards to assess the potential societal impact of AI systems before deployment.
According to Paul Sebastien Ziegler, CEO of cybersecurity training company Reflare, “AI provides an incredible wealth of features, along with an immense and uncalculatable risk surface.”
To help organisations navigate the new threats posed by AI, Reflare created this helpful guide. NIST and BSI have both created robust AI risk management frameworks that are also worth reviewing.
4. An Agile Approach to Opportunity Assessment and Exploitation
AI is evolving rapidly, and organisations must be agile in assessing and leveraging new opportunities. This includes:
- Continuous Learning and Experimentation: Encourage a culture of innovation by allowing teams to experiment with AI technologies, fail fast, and iterate quickly.
- Scalability Considerations: Ensure that AI solutions are scalable and adaptable to changing business needs.
- Industry Benchmarking: Regularly evaluate AI trends and best practices from leading industry players to stay ahead of the curve.
5. Reporting and Accountability
Accountability and transparency in AI usage are crucial for building trust among stakeholders. Organisations should:
- Develop Clear Reporting Mechanisms: Implement structured reporting on AI performance, risks, and ethical considerations.
- Define Metrics for Success: Establish measurable KPIs to assess the impact and effectiveness of AI initiatives.
- Ensure Regulatory Compliance: Regularly audit AI systems to ensure they comply with industry standards and legal requirements.
A strong AI strategy goes beyond policy creation - it involves clear objectives, solid governance, robust risk management, agile adaptation, and transparent reporting.
Organisations that integrate these elements into their AI strategy will be well-positioned to harness the power of AI responsibly while driving meaningful business outcomes. By taking a structured and ethical approach, companies can unlock AI’s full potential while mitigating risks and fostering stakeholder trust.