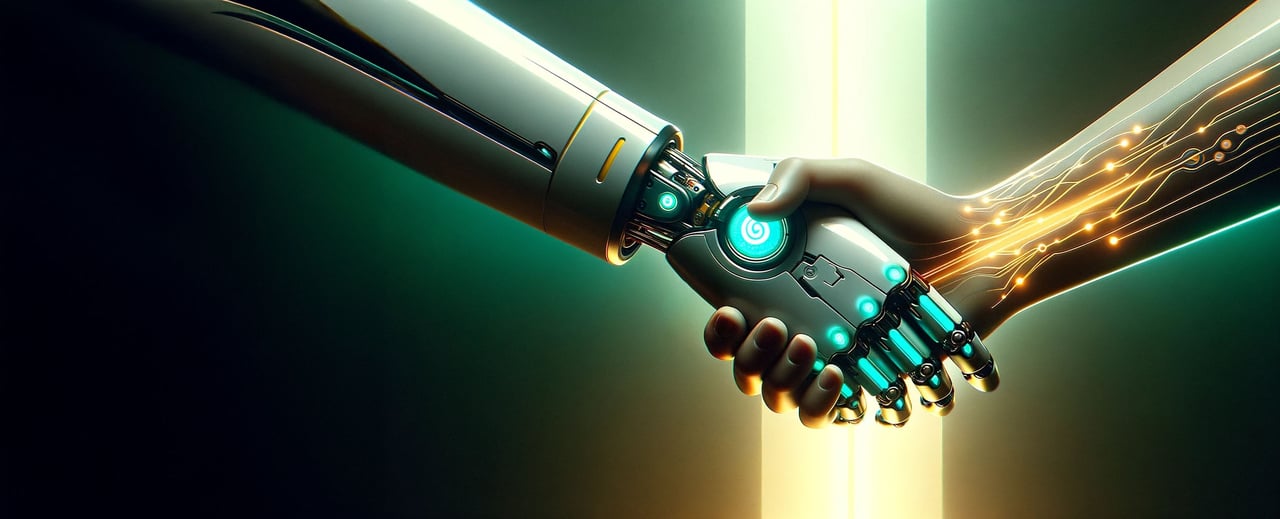
Strivenn Thinking
Governing AI Responsibly
By Matt Wilkinson
AI is being woven into the fabric of decision-making - influencing outcomes in everything from how marketers generate content to how cancer is diagnosed. That’s why last week, I happily took advantage of a fantastic AI Management Standards training course organised by BSI and Innovate UK as part of the BridgeAI scheme and gain the AI Management Practitioner professional qualification.
The training course is centred around the world’s first Artificial Intelligence Management System (AIMS) framework ISO/IEC 42001:2023. The course represents a shift in how we think about the ethics, risks, and the societal responsibilities of AI.
From Compliance to Conscious Design
The reflex to manage AI often begins with regulation. But regulations lag innovation. Standards like ISO 42001 and 23894 bridge this gap, providing a structured yet flexible foundation for organisations to build AI Management Systems (AIMS) that don’t just respond to policy - but anticipate it.
ISO 42001 helps embed AI governance across the organisation, aligning intent, oversight, and operations. Meanwhile, ISO 23894 focuses on risk management, helping organisations map out where AI can go wrong - not just technically but socially.
The key principles include:
- Transparency: AI decisions must be explainable and understandable to stakeholders.
- Accountability: Organizations must justify and document AI-related decisions.
- Fairness: Prevents bias and discrimination in automated decision-making.
- Security & Safety: Protects AI systems from threats and ensures they do not harm people or property.
- Data Quality & Privacy: Maintains the integrity and privacy of data used in AI systems.
- Reliability: Ensures AI systems function safely and as intended
Plan-Do-Check-Act
Central to the approach is Clause 8 of ISO/IEC 42001 embeds the Plan-Do-Check-Act (PDCA) cycle at the core of operationalising an AI Management System (AIMS).
Rather than treating governance as a static checklist, the PDCA model reinforces continuous improvement and responsiveness.
In the planning phase, organisations define objectives, identify AI-specific risks, and establish controls tailored to the intended uses of AI systems.
The doing phase involves implementing those controls and processes across the AI lifecycle - often requiring cross-functional collaboration between technical, legal, and ethical domains.
The check phase emphasises performance evaluation, including audits and incident tracking, to assess the effectiveness of safeguards.
Finally, the act phase ensures learnings are fed back into the system, closing the loop through adaptation and refinement.
In this way, Clause 8 transforms AI governance into a living practice - capable of evolving alongside the technology it seeks to manage.
The Ethical Core of AI Governance
At its heart, governance isn’t about limiting AI - it’s about directing it responsibly. Ethics cannot be outsourced to legal or technical teams alone. They must be part of the design process, the leadership culture, and the strategic vision.
Training programs rooted in these standards offer more than knowledge - they provide a shared language and set of tools to:
- Align AI systems with organisational values and public expectations.
- Build transparent impact assessments that go beyond cost-benefit.
- Foster a culture of reflection around the use and misuse of AI.
Bias Isn’t a Bug - It’s a Governance Issue
Bias in AI doesn’t just happen in code. It’s a byproduct of systemic blind spots - in the data we choose, the questions we ask, and the outcomes we optimise for. An AI system trained on skewed historical data may reinforce injustice under the guise of efficiency – one only has to watch the Netflix Documentary Coded Bias to see how unintentionally biased input data can cause unintentional harms.
Addressing challenges like this upfront is where structured risk frameworks shine. ISO/IEC 23894 pushes practitioners to ask:
- Who might this system unintentionally disadvantage?
- What are the social costs if it fails?
- How do we balance accuracy with fairness?
By formalising such questions, organisations move from reactive patchwork to proactive design.
Why This Matters Now
With regulations like the EU AI Act looming and public trust in tech wavering, organisations face a clear inflection point. Responsible AI is no longer a differentiator - it’s a necessity.
The path forward involves not only innovating faster, but governing smarter. That means equipping teams with the capacity to ask tough questions, anticipate risk, and translate ethical intent into operational practice.